“Real-Time Escape Route Generation in Low Visibility Environments” details a comprehensive system for navigation and path planning in the complex environment of a smoky structure fire. This is achieved through the use of a swarm of multimodal LiDAR-SONAR perception systems (regulated by a particulate matter sensor) that quickly produce a map of the building, resolve its geometry into a visibility graph populated with safety information, and use a custom reinforcement learning algorithm (LFA-NPG) to navigate the space.
Abstract:
Structure fires are responsible for the majority of fire-related deaths nationwide. In order to assist with the rapid evacuation of trapped people, this paper proposes the use of a system that determines optimal search paths for firefighters and exit paths for civilians in real time based on environmental measurements. Through the use of a LiDAR mapping system evaluated and verified by a trust range derived from sonar and smoke concentration data, a proposed solution to low visibility mapping is tested. These independent point clouds are then used to create distinct maps, which are merged through the use of a RANSAC based alignment methodology and simplified into a visibility graph. Temperature and humidity data are then used to label each node with a danger score, creating an environment tensor. After demonstrating how a Linear Function Approximation based Natural Policy Gradient RL methodology outperforms more complex competitors with respect to robustness and speed, this paper outlines two systems (savior and refugee) that process the environment tensor to create safe rescue and escape routes, respectively.
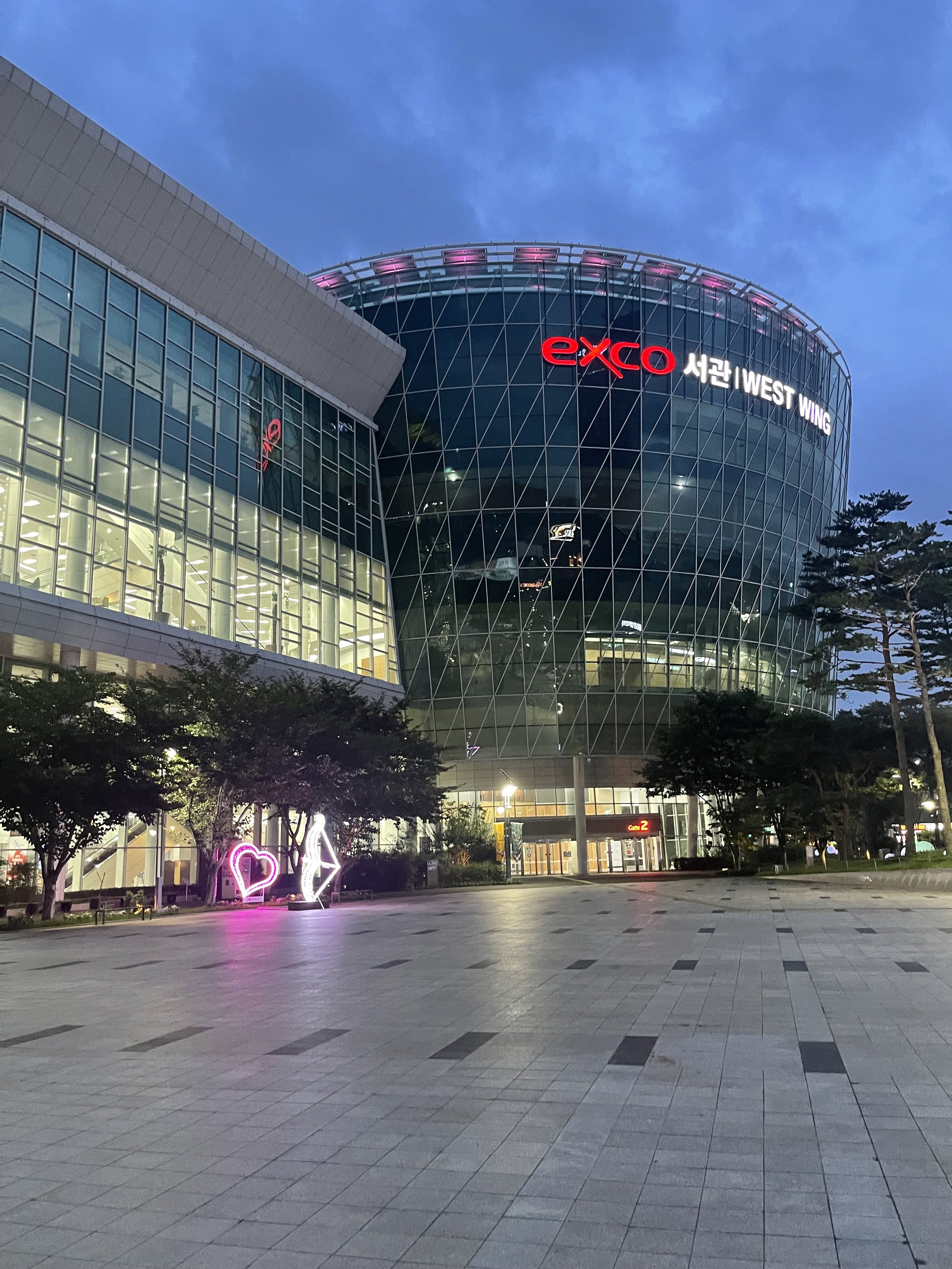
Where did I Present?
I published my work at the Robotics: Science and Systems Conference in Daegu, South Korea, in the workshop “Taking Mobile Manipulators into the Real World”.
Physical Design
My physical data collection device was a custom drone made out of carbon fiber and PETG plastic. The drone itself is agile, with a thrust/weight ratio of 2.8. My perception module initially just had a 360 degree RPLiDAR sensor, but afterwards I extended the perception capabilities by adding four sonar sensors for higher fidelity readings. The housing for the sensors, as well as the battery case and legs were custom 3D printed, with topology optimization used in CAD to streamline the geometry to yield the maximum performance for the part’s weight.
The control stack of my physical drone uses a Raspberry Pi running Ubuntu with ROS that interfaces directly with the sensor components, alongside a Pixhawk Flight Control Unit to oversee the autopilot control and movement of the drone. To connect to the drone, I used the radio antenna to transmit data from the server. To display data, I created a virtual server on a docker container with a Linux VM client with ROS on my laptop that could pull data from the drone. This also enabled me to integrate my physical setup into simulations, and log data for model training.
Maps & Algorithms
I recorded data across a mix of physical and simulated devices. I began by modeling the dynamics of my drone in matlab, so that its control systems performed as intended. I then designed a physical drone, using LiDAR rangefinders and sonar scanners to test its mapping capacity in smoke. I created “smoke” using a smoke machine (replacing the particulate matter sensor with a comparable alcohol sensor) during testing to simulate the challenging conditions of a fire, enabling the system to collect accurate data on the adversarial noise parameter. In addition, I collected temperature and particulate matter concentration to determine danger scores.Various SLAM calculations, including GMapping and other methodologies, were crucial in the efficient gathering and recording of data in smoky environments. After determining the performance of my physical drone, I created software simulations in code to focus on key aspects of drone performance, and simulated other drones in a swarm. My emphasis on the collection of real-time data through a fleet of autonomous drones and the comprehensive mapping of the environment was essential in ensuring the accuracy and reliability of the proposed system.
My data processing was split into two phases: once for my perception module, and again for my Reinforcement Learning algorithm. After examining the data I collected on LiDAR and SONAR performance in smoke, I decided to use a probabilistic measure of node traversability. This had two benefits: Firstly, since it was a continuous form of data, I could use interpolation to fill in areas that the mapping system had not yet reached. Secondly, it enabled quick error correction. I also prioritized complexity reduction when designing my map reintegration algorithm. I used a correlative scan matcher to sync the data across the swarm, and then converted the map into a visibility graph with one meter node separation. This enabled navigation algorithms to run much faster, and also made the map much easier to read.
Motivation
My passion for robotics and a strong sense of social responsibility drove my interest in this research. Learning about robotics during the COVID-19 pandemic and witnessing the impact of wildfires in California motivated me to use my expertise to create solutions benefiting my community. My focus was on devising efficient systems for emergency operations, prioritizing safe and swift navigation over absolute optimization. I established research goals centered on precise perception and fast navigation, drawing insights from various sources like online courses and papers. Witnessing the challenges faced by my community inspired me to extend my prior work on Reinforcement Learning with a high fidelity computer vision system to improve firefighter response.